Benefits of Machine Learning: How It Improves Our World
Published: 05 Feb 2025
Machine learning (ML) is transforming industries worldwide, from healthcare to finance. In fact, it’s estimated that by 2030, the global impact of AI and ML will exceed $15 trillion. With its ability to analyze large datasets and make predictions, ML is reshaping how businesses make decisions, personalize services, and automate tasks, unlocking numerous benefits across various sectors.
Introduction
Machine learning (ML) is a branch of artificial intelligence that allows machines to learn from data without being explicitly programmed. In simple terms, it means that instead of telling a computer what to do step-by-step, we feed it data, and it learns patterns to make decisions or predictions on its own. For example, Netflix uses ML to recommend shows or movies based on your previous watching habits. The more you watch, the better it gets at suggesting content you’ll likely enjoy.
Machine learning plays a key role in a wide range of industries today, including healthcare, finance, entertainment, and more. It helps businesses and organizations solve complex problems, make smarter decisions, and improve services. In this article, we’ll explore the benefits of machine learning and how it’s transforming the way we live and work.
Core Benefits of Machine Learning
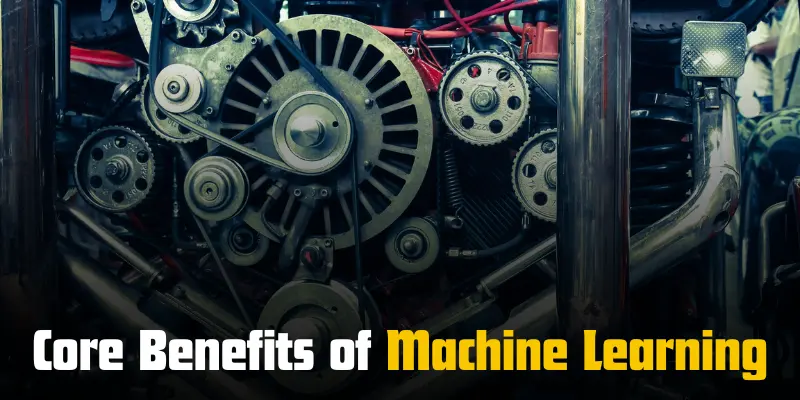
Benefit 1: Automation of Repetitive Tasks
- Save Time and Effort: ML automates repetitive tasks, reducing the need for human intervention.
- Efficiency Boost: Tasks that once took hours can now be completed in seconds.
- Example: Email Filtering
- ML automatically sorts emails into your inbox and spam folders.
- It learns which emails are important and which are not based on patterns from previous interactions.
Benefit 2: Improved Decision-Making
- Better Predictions: ML helps businesses predict future trends based on past data.
- Increased Accuracy: It reduces the risk of human errors in decision-making.
- Example: Predictive Analytics in Sales or Marketing
- Businesses use ML to understand customer behavior and predict what products or services they might buy.
- This helps them target the right audience with personalized offers.
Benefit 3: Personalization
- Tailored Experiences: ML personalizes content and product recommendations based on individual preferences.
- User Satisfaction: It makes services more relevant and enjoyable for users.
- Example: Personalized Recommendations
- Amazon suggests products based on your past purchases.
- Spotify creates personalized playlists based on what you’ve listened to before, making music discovery easier.
Benefit 4: Handling Large Datasets
- Efficient Data Processing: ML can analyze massive amounts of data much faster than humans.
- Uncover Hidden Insights: It finds patterns in big data that would be impossible for a human to notice.
- Example: Healthcare Applications
- ML is used to analyze medical images like X-rays or MRIs, helping doctors detect diseases like cancer earlier.
- It also helps in predicting patient outcomes by analyzing large datasets of health records.
Benefit 5: Continuous Improvement
- Learning from Data: ML models get better over time as they are exposed to more data.
- Adaptability: They can adjust and improve based on new information.
- Example: Self-Driving Cars
- Self-driving cars use ML to learn from real-world driving experiences.
- The more they drive, the better they get at understanding road conditions, avoiding obstacles, and making decisions in real time.
Real-Life Applications of Machine Learning
Healthcare
Machine learning is revolutionizing the healthcare industry by helping doctors diagnose diseases more quickly and accurately. For example, AI-powered tools can analyze medical images like X-rays and MRIs to detect tumors that might be missed by the human eye. This ability to spot potential health issues early can save lives by enabling timely treatments.
Tip: Machine learning helps provide early warnings for health conditions, leading to quicker interventions and better patient outcomes.
E-Commerce
In the e-commerce world, machine learning plays a huge role in personalizing customer experiences. Online stores use ML algorithms to recommend products based on your past purchases or browsing behavior. So, when you shop on Amazon, the suggestions you see are powered by machine learning, designed to match your preferences.
Tip: By personalizing recommendations, ML boosts sales and improves customer satisfaction, creating a more enjoyable shopping experience.
Finance
Machine learning is helping the finance industry tackle fraud in real-time. Banks and financial institutions use ML to monitor transactions and detect unusual activities on accounts—like sudden large withdrawals or purchases in unfamiliar locations. This helps prevent fraudulent activities before they cause major losses.
Tip: With ML’s fraud detection systems, both consumers and businesses are protected from financial theft, creating a safer financial environment.
Entertainment
Streaming platforms like Netflix and YouTube rely on machine learning to recommend shows and videos tailored to your tastes. By analyzing your viewing habits, ML algorithms suggest new content you’re likely to enjoy. This makes your entertainment experience smoother and more engaging.
Tip: ML enhances user experiences by offering relevant content recommendations, making it easier to discover new shows or videos you wouldn’t have found otherwise.
How Machine Learning Works (Basic Overview)
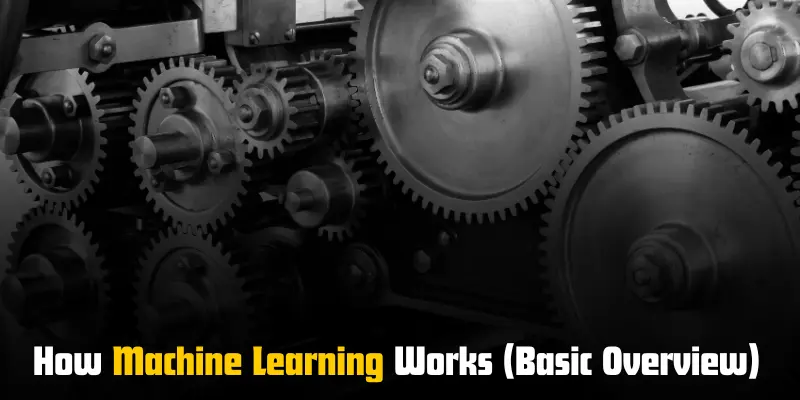
Machine learning (ML) allows computers to learn from data and make predictions or decisions without being explicitly programmed. Here’s a simple breakdown of how ML works:
Training the Model
- What it Means: This is the first step where the model learns from data by recognizing patterns.
- How it Works: We feed the model large amounts of data so it can understand features or characteristics.
- Goal: The goal is to create a model that can make accurate predictions or decisions when given new data.
Example:
To train a model to recognize cats in pictures, we show it thousands of images labeled as either “cat” or “not cat.” Over time, the model learns what features make up a cat (like shape, ears, and whiskers).
Learning from Data
- What it Means: In this step, the model “learns” by analyzing the data and finding hidden patterns.
- How it Works: ML algorithms search through the data to identify trends and correlations that can be used for future decisions.
- Goal: The model continues to improve its understanding as it processes more data.
Example:
A music recommendation system learns your taste by tracking the songs you listen to. Over time, it gets better at recommending new music you might like based on what you’ve enjoyed in the past.
Making Predictions
- What it Means: After being trained, the model uses its learning to make predictions or decisions.
- How it Works: The trained model applies the patterns it has learned to new, unseen data to provide an outcome.
- Goal: The goal is for the model to be able to predict outcomes based on previous learnings.
Example:
Google Translate uses past data from many languages to predict the best translation when you enter a sentence. The more data it processes, the better it gets at translating accurately.
Challenges of Machine Learning
While machine learning (ML) offers tremendous benefits, it comes with its own set of challenges. Here are some of the main issues faced when implementing ML:
Data Quality
- What it Means: For machine learning models to be accurate, they need high-quality data.
- How it Works: If the data used to train the model is inaccurate, incomplete, or noisy, the results will be poor and unreliable.
- Goal: Clean and accurate data ensures the model learns correctly, leading to better predictions.
Tip:
Always ensure that the data used for training is free from errors, consistent, and representative of the problem you’re trying to solve. Regularly clean and update data to improve accuracy.
Complexity
- What it Means: Some machine learning models, especially deep learning models, can be difficult to understand and explain.
- How it Works: Many ML models, like neural networks, are highly complex and act as “black boxes,” meaning their decision-making process isn’t clear to humans.
- Goal: While these models can be highly effective, the lack of transparency makes it harder to trust and explain their decisions.
Example:
AI systems used in hiring processes might recommend candidates based on patterns, but it’s often unclear why the system chooses one candidate over another. This can be frustrating for decision-makers trying to understand the model’s reasoning.
Bias in Data
- What it Means: Machine learning models can inherit biases from the data they are trained on, leading to unfair or skewed results.
- How it Works: If the training data contains biases (e.g., gender, race, or socioeconomic status), the model may unintentionally favor certain groups over others.
- Goal: The goal is to ensure that the model’s outcomes are fair and equitable for everyone.
Tip:
Ensure your data is diverse and balanced. Carefully review the data for any inherent biases and make adjustments as needed. This helps avoid unfair or discriminatory results in the predictions.
Conclusion
So guys, in this article, we’ve covered the benefits of machine learning in detail. From automating tasks to providing personalized experiences, ML is making a huge impact. If you’re looking to leverage this powerful tool, I recommend starting with simple, beginner-friendly resources to get your feet wet. Don’t wait for the future—get ahead of the curve today! Ready to start? Find a project and see how ML can make a difference for you.
FAQs About the Benefits of Machine Learning
Machine learning is a type of artificial intelligence where computers learn from data to make decisions without being explicitly programmed. It works by recognizing patterns in data and using them to make predictions. Over time, the system improves as it processes more information.
In traditional programming, humans write specific instructions for the computer to follow. In machine learning, the computer learns by analyzing data and identifying patterns on its own. The machine essentially “learns” how to make decisions based on the data it receives.
Some common examples of machine learning include Netflix recommending movies, email filtering out spam, and voice assistants like Siri understanding your commands. These are all powered by ML algorithms that learn from user interactions and data to improve over time.
Yes! Small businesses can benefit from machine learning by using it for customer service, marketing, and sales predictions. Even simple ML tools can help with tasks like automating responses or personalizing marketing campaigns based on customer behavior.
Not at all! There are many beginner-friendly tools and resources available to help anyone start with machine learning. You can learn the basics and start experimenting with pre-built models without needing an advanced degree in AI or coding.
Machine learning works best with large, clean, and accurate datasets. The data should be relevant to the problem you’re trying to solve and should include examples that help the model learn patterns. For instance, a recommendation system needs data on past preferences to suggest new items effectively.
Machine learning improves by continuously learning from new data. The more data the system receives, the better it can identify patterns and make predictions. This process of learning and refining helps it provide more accurate results as time goes on.
Yes, machine learning is increasingly used in healthcare to analyze medical data, predict disease outcomes, and assist in diagnosing conditions like cancer. ML can help doctors make faster and more accurate decisions, improving patient care.
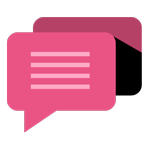
- Be Respectful
- Stay Relevant
- Stay Positive
- True Feedback
- Encourage Discussion
- Avoid Spamming
- No Fake News
- Don't Copy-Paste
- No Personal Attacks
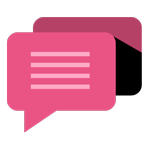
- Be Respectful
- Stay Relevant
- Stay Positive
- True Feedback
- Encourage Discussion
- Avoid Spamming
- No Fake News
- Don't Copy-Paste
- No Personal Attacks